7 enterprise data strategy trends
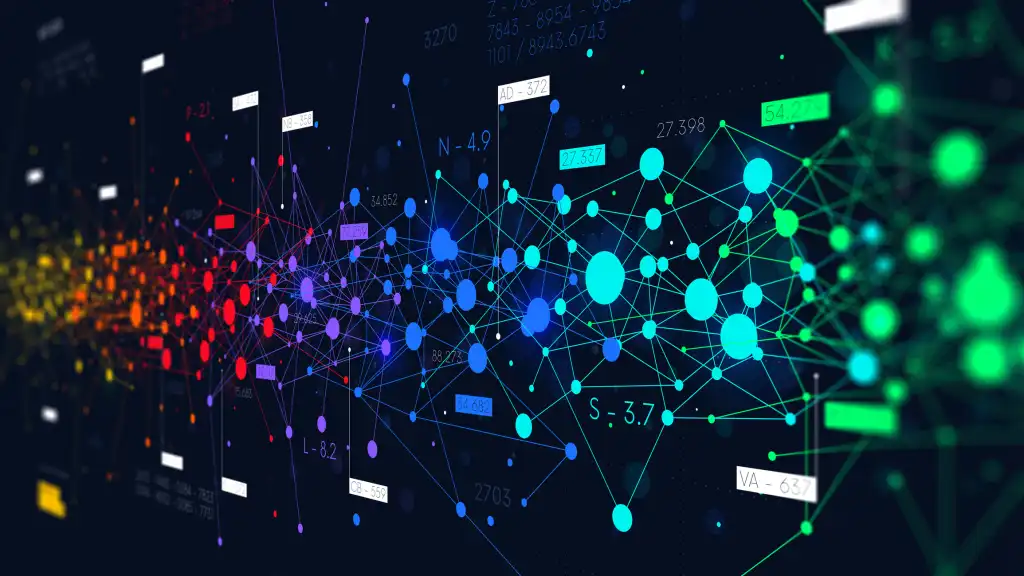
A strong, up-to-date data strategy is fundamental to your enterprise’s long-term success. In light of ongoing changes, it will probably need some serious updating.
Every enterprise needs a data strategy that clearly defines the technologies, processes, people, and rules needed to safely and securely manage its information assets and practices.
As with just about everything in IT, a data strategy must evolve over time to keep pace with evolving technologies, customers, markets, business needs and practices, regulations, and a virtually endless number of other priorities.
Here’s a quick rundown of seven major trends that will likely reshape your organization’s current data strategy in the days and months ahead.
1. Real-time data gets real — as does the complexity of dealing with it
CIOs should prioritize their investment strategy to cope with the growing volume of complex, real-time data that’s pouring into the enterprise, advises Lan Guan, global data and AI lead at business consulting firm Accenture.
Guan believes that having the ability to harness data is non-negotiable in today’s business environment. “Unique insights derived from an organization’s data constitute a competitive advantage that’s inherent to their business and not easily copied by competitors,” she observes. “Failing to meet these needs means getting left behind and missing out on the many opportunities made possible by advances in data analytics.”
The next step in every organization’s data strategy, Guan says, should be investing in and leveraging artificial intelligence and machine learning to unlock more value out of their data. “Initiatives such as automated predictive maintenance on machinery or workforce optimization through operational data are only a few of the many opportunities enabled by the pairing of a successful data strategy with the impactful deployment of artificial intelligence.”
2. In-house data access demands take center stage
CIOs and data leaders are facing a growing demand for internal data access. “Data is no longer just used by analysts and data scientists,” says Dinesh Nirmal, general manager of AI and automation at IBM Data. “Everyone in their organization — from sales to marketing to HR to operations — needs access to data to make better decisions.”
The downside is that providing easy access to timely, relevant data has become increasingly challenging. “Despite massive investments, the data landscape within enterprises is still overly complex, spread across multiple clouds, applications, locations, environments, and vendors,” Nirmal says.
As a result, a growing number of IT leaders are looking for data strategies that will allow them to manage the massive amounts of disparate data located in silos without introducing new risk and compliance challenges. “While the need for data access internally is rising, [CIOs] also have to keep pace with rapidly evolving regulatory and compliance measures, like the EU Artificial Intelligence Act and the newly released White House Blueprint for an AI Bill of Rights,” Nirmal says.
3. External data sharing gets strategic
Data sharing between business partners is becoming far easier and much more cooperative, observes Mike Bechtel, chief futurist at business advisory firm Deloitte Consulting. “With the meaningful adoption of cloud-native data warehouses and adjacent data insights platforms, we’re starting to see interesting use cases where enterprises are able to braid their data with counterparties’ data to create altogether new, salable, digital assets,” he says.
Bechtel envisions an upcoming sea change in external data sharing. “For years, boardroom and server room folks alike have talked abstractly about the value of having all this data, but the geeks among us have known that the ability to monetize that data required it to be more liquid,” he says. “Organizations may have petabytes of interesting data, but if it’s calcified in an aging on-premises warehouse, you’re not going to be able to do much with it.”
4. Data fabric and data mesh adoption rises
Data fabric and data mesh technologies can help organizations squeeze the maximum value out of all the elements in a technical stack and hierarchy in a practical and usable manner. “Many enterprises still utilize legacy solutions, old and new technologies, inherited policies, processes, procedures, or approaches, but wrestle with having to blend it all within a new architecture that enables more agility and speed,” says Paola Saibene, principal consultant at IT advisory firm Resultant.
Mesh enables an organization to draw the information and insights it needs from the environment in its current state without having to radically change it or massively disrupt it. “This way, CIOs can take advantage of [tools] they already have, but add a layer on top that allows them to make use of all those assets in a modern and fast way,” Saibene explains.
Data fabric is an architecture that enables the end-to-end integration of various data pipelines and cloud environments through the use of intelligent and automated systems. The fabric, especially at the active metadata level, is important, Saibene notes. “Interoperability agents will make it look like everything is incredibly well-connected and has been intentionally architected that way,” she says. “As such, you’re able to gain all the insights you need while avoiding having to overhaul your environment.”
5. Data observability becomes business-critical
Data observability extends the concept of data quality by closely monitoring data as it flows in and out of the applications. The approach provides business-critical insights into application information, schema, metrics, and lineage, says Andy Petrella, founder of data observability provider, Kensu, and the author of Fundamentals of Data Observability (O’Reilly, 2022).
A key data observability attribute is that it acts on metadata, providing a safe way to monitor data directly within applications. As sensitive data leaves the data pipeline; it’s collected by a data observability agent, Petrella says. “Thanks to this information, data teams can troubleshoot data issues faster and prevent them from propagating, lowering maintenance costs, restoring trust in data, and scaling up value creation from data,” he adds.
Data observability creates an entirely new solution category, Petrella claims. “CIOs should first understand the different approaches to observing data and how it differs from quality management,” he notes. They should then identify the stakeholders in their data team, since they will be responsible for adopting observability technology.
An inability to improve data quality will likely hinder data team productivity while decreasing data trust across the entire data chain. “In the long term, this could push data activities into the background, impacting the organization’s competitiveness and ultimately its revenue,” Petrella states.
IT leaders are contending with soaring complexity and unfathomable volumes of data spread across the technology stack, observes Gregg Ostrowski, executive CTO of Cisco AppDynamics. “They’re having to integrate a massively expanding set of cloud-native services with existing on-premise technologies,” he notes. “From a data strategy perspective, the biggest trend is the need for IT teams to get clear visualization and insight in their applications irrespective of domain, whether on-premises, in the cloud or hybrid environments.”
6. ‘Data as a product’ begins delivering business value
Data as a product is a concept that aims to solve real-world business problems through the use of blended data captured from many different sources. “This capture-and-analyze approach provides a new level of intelligence for companies that can result in a real, bottom-line impact,” says Irvin Bishop, Jr., CIO at Black & Veatch, a global engineering, procurement, consulting, and construction company.
Understanding how to harvest and apply data can be a game-changer in many ways, Bishop states. He reports that Black & Veatch is working with clients to develop data product roadmaps and establish relevant KPIs. “One example is how we utilize data within the water industry to better manage the physical health of critical infrastructure,” he notes. “Data gives our water clients the ability to predict when a piece of equipment will likely need to be replaced and what type of environmental impact it can withstand based on past performance data.” Bishop says that the approach gives participating clients more control over service reliability and their budgets.
7. Cross-functional data product teams arise
As organizations begin treating data as a product, it’s becoming necessary to establish product teams that are connected across IT, business, and data science sectors, says Traci Gusher, data and analytics leader at business advisory firm EY Americas.
Data collection and management shouldn’t be classified as just another project, Gusher notes. “Data needs to be viewed as a fully functional business area, no different than HR or finance,” she claims. “The move to a data product approach means your data will be treated just like a physical product would be — developed, marketed, quality controlled, enhanced, and with a clear tracked value.”